Dr Maryam Haghighat
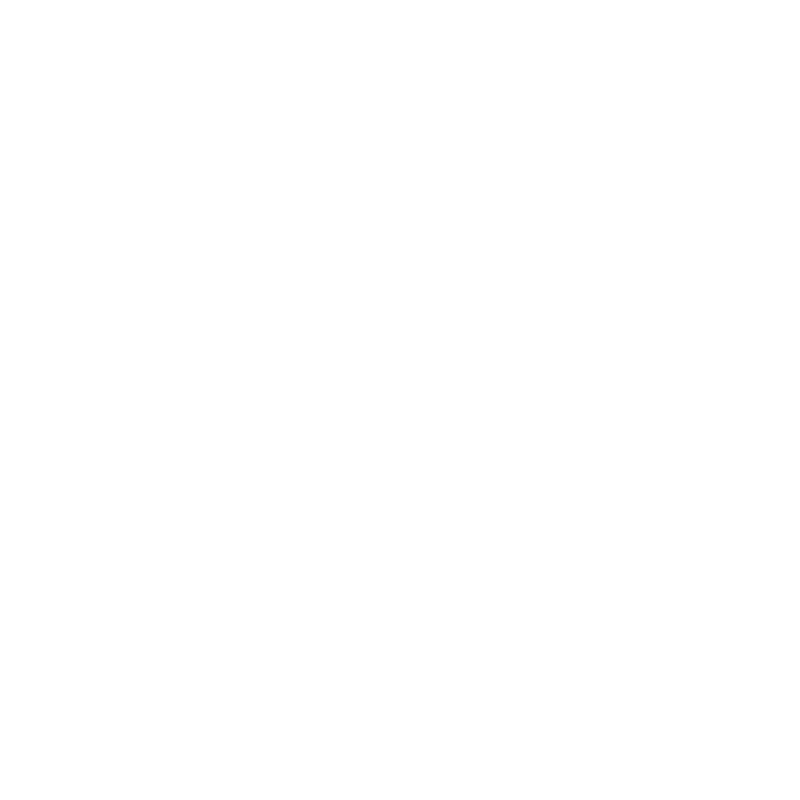
Faculty of Engineering,
School of Electrical Engineering & Robotics
Biography
Maryam Haghighat is a lecturer at the QUT School of Electrical Engineering and Robotics. She received her PhD from the UNSW School of Electrical Engineering and Telecommunications, Australia. Her doctoral research in image processing at the "Interactive Visual Media Processing" lab, was recognised by the 2020 UNSW Award for Outstanding Doctoral Thesis.Following her PhD, Maryam joined the Big Data Institute (BDI), Department of Engineering Science, University of Oxford, UK, as a postdoctoral researcher from 2020 to 2022. As a key member of PathLAKE project funded by InnovateUK, she led development of machine learning algorithms for medical image analysis.
In 2022, Maryam conducted research jointly across the CSIRO Machine Learning and Artificial Intelligence Future Science Platform and the Mineral Resources Business Unit in hyperspectral deep learning.
Maryam is currently leading projects in machine learning and computer vision with applications in robotics, healthcare, and remote sensing.
Personal details
Positions
- Lecturer
Faculty of Engineering,
School of Electrical Engineering & Robotics
Keywords
Machine Learning, Computer Vision, Deep Learning, Artificial Intelligence, Robotics, Signal Processing, Image Processing
Qualifications
- Doctor of Philosophy (University of New South Wales)
Teaching
- EGH444, Digital Signals and Image Processing, Lecturer and Unit Coordinator
- ENN585, Advanced Machine Learning, Lecturer
- ENN595-1/2, Master of Robotics and AI Research Project , Lecturer and Unit Coordinator
Publications
- Mohamed, S., Haghighat, M., Fernando, T., Sridharan, S., Fookes, C. & Moghadam, P. (2024). FactoFormer: Factorized Hyperspectral Transformers with Self-Supervised Pre-Training. IEEE Transactions on Geoscience and Remote Sensing, 62. https://eprints.qut.edu.au/245518
- Ramezani, M., Griffiths, E., Haghighat, M., Pitt, A. & Moghadam, P. (2023). Deep Robust Multi-Robot Re-Localisation in Natural Environments. Proceedings of the 2023 IEEE/RSJ International Conference on Intelligent Robots and Systems (IROS), 3322–3328. https://eprints.qut.edu.au/246126
- Haghighat, M., Browning, L., Sirinukunwattana, K., Malacrino, S., Khalid Alham, N., Colling, R., Cui, Y., Rakha, E., Hamdy, F., Verrill, C. & Rittscher, J. (2022). Automated quality assessment of large digitised histology cohorts by artificial intelligence. Scientific Reports, 12(1). https://eprints.qut.edu.au/237203
- Ali, S., Bailey, A., Ash, S., Haghighat, M., Allan, P., Ambrose, T., Arancibia-Cárcamo, C., Barnes, E., Bird-Lieberman, E., Bornschein, J., Brain, O., Collier, J., Culver, E., Geremia, A., George, B., Howarth, L., Jones, K., Klenerman, P., Palmer, R., Powrie, F., Rodrigues, A., Satsangi, J., Simmons, A., Travis, S., Uhlig, H., Walsh, A., Leedham, S., Lu, X., East, J., Rittscher, J., Braden, B. & other, a. (2021). A Pilot Study on Automatic Three-Dimensional Quantification of Barrett's Esophagus for Risk Stratification and Therapy Monitoring. Gastroenterology, 161(3), 865–878.e8. https://eprints.qut.edu.au/237155
- Chatrian, A., Colling, R., Browning, L., Alham, N., Sirinukunwattana, K., Malacrino, S., Haghighat, M., Aberdeen, A., Monks, A., Moxley-Wyles, B., Rakha, E., Snead, D., Rittscher, J. & Verrill, C. (2021). Artificial intelligence for advance requesting of immunohistochemistry in diagnostically uncertain prostate biopsies. Modern Pathology, 34(9), 1780–1794. https://eprints.qut.edu.au/237216
- Haghighat, M., Mathew, R. & Taubman, D. (2020). Rate-distortion driven decomposition of multiview imagery to diffuse and specular components. IEEE Transactions on Image Processing, 29, 5469–5480. https://eprints.qut.edu.au/237402
- Haghighat, M., Mathew, R., Naman, A. & Taubman, D. (2019). Illumination Estimation and Compensation of Low Frame Rate Video Sequences for Wavelet-Based Video Compression. IEEE Transactions on Image Processing, 28(9), 4313–4327. https://eprints.qut.edu.au/237413
- Haghighat, M., Mathew, R., Naman, A., Young, S. & Taubman, D. (2018). Rate-distortion optimized illumination estimation for wavelet-based video coding. Proceedings of the 2018 IEEE International Conference on Acoustics, Speech and Signal Processing (ICASSP 2018), 1213–1217. https://eprints.qut.edu.au/237564
QUT ePrints
For more publications by Maryam, explore their research in QUT ePrints (our digital repository).
Supervision
Looking for a postgraduate research supervisor?
I am currently accepting research students for Honours, Masters and PhD study.
- Re-localisation in natural environments
- Enhancing 3D visual understanding through multimodal data fusion
You can browse existing student topics offered by QUT or propose your own topic.
Current supervisions
- PhD, Principal Supervisor
Other supervisors: Professor Clinton Fookes, Associate Professor Simon Denman - Deep Spatial-Spectral Representation Learning for Hyperspectral Data
PhD, Associate Supervisor
Other supervisors: Professor Sridha Sridharan, Adjunct Professor Peyman Moghadam, Professor Clinton Fookes, Dr Tharindu Fernando Warnakulasuriya
The supervisions listed above are only a selection.