Professor Niko Suenderhauf
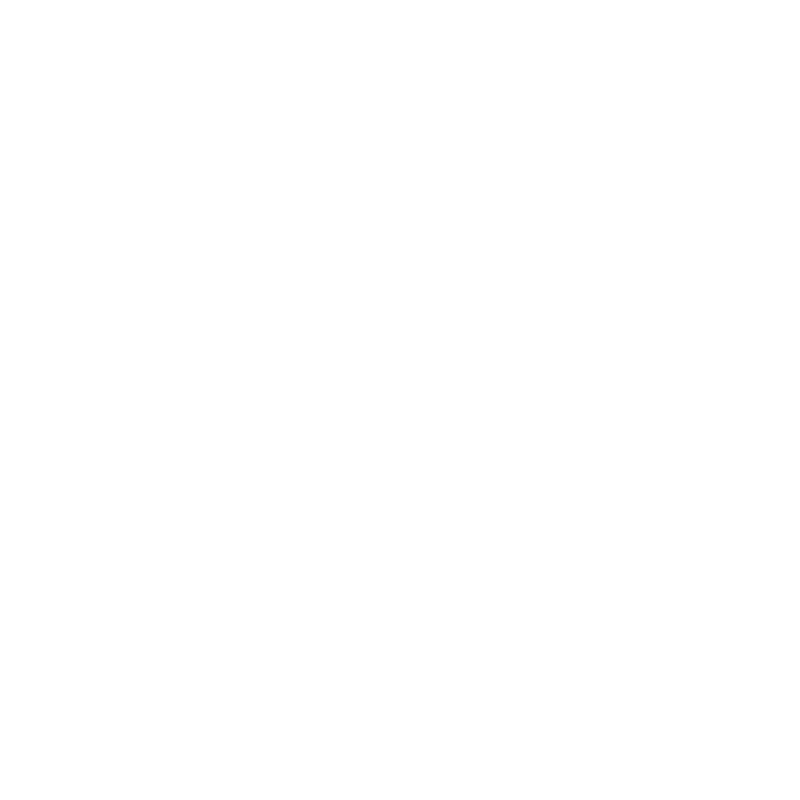
Faculty of Engineering,
School of Electrical Engineering & Robotics
Biography
Professor Niko Suenderhauf is Deputy Director (Research) of the ARC Research Hub (ITRH) in Intelligent Robotic Systems for Real-Time Asset Management, and lead-CI for an ARC Discovery Project (2022-25). He is Chief Investigator and member of the Executive Committee of the QUT Centre for Robotics (QCR) and leads the Visual Learning and Understanding program. He has been Acting Joint Director of the QUT Centre for Robotics between October 2022 and March 2023. He was QCR's acting Deputy Director Feb-July 2021. Between 2017 and 2020 Niko was Chief Investigator and Project Leader of the Australian Centre of Excellence for Robotic Vision (ACRV).Niko conducts research in robotic vision and robotic learning, at the intersection of robotics, computer vision, machine learning and AI. His research is driven by the question of how robots can learn to perform complex tasks. Solving this problem requires robust perception, scene understanding, high-level planning and reasoning, and the capability to interact with objects and humans.
Niko's research group develops innovative ways of incorporating Large Language Models into robotics, leveraging their abilities for high-level planning and common-sense reasoning. His group also explores the utility of other foundation models, such as vision-language models, for robotic perception, scene understanding, learning, and mapping.
Niko is very interested in questions about the reliability, safety and robustness of machine learning for real-world applications.
Prof Suenderhauf regularly organises workshops at leading robotics and computer vision conferences. He was was co-chair of the IEEE Robotics and Automation Society Technical Committee on Robotic Perception (2020-2022), was a member of the editorial board for the International Journal of Robotics Research (IJRR, 2019-2022), and Associate Editor for the IEEE Robotics and Automation Letters journal (RA-L) from 2015 to 2019. Niko served as AE for the IEEE International Conference on Robotics and Automation (ICRA) 2018 and 2020.
As an educator at QUT, Niko teaches Robotic Vision (ENN583) and Advanced Machine Learning (ENN585) in the Master's of Robotics and AI. He previously enjoyed teaching Introduction to Robotics (EGB339), Mechatronics Design 3 (EGH419), as well as Digital Signals and Image Processing (EGH444) to the undergraduate students in the Electrical Engineering degree.
Niko received his PhD from Chemnitz University of Technology, Germany in 2012. In his thesis, Niko focused on robust factor graph-based models for robotic localisation and mapping, as well as general probabilistic estimation problems, and developed the mathematical concepts of Switchable Constraints. After two years as a Research Fellow in Chemnitz, Niko joined QUT as a Research Fellow in March 2014, before being appointed to a tenured Lecturer position in 2017.
Personal details
Positions
- Professor
Faculty of Engineering,
School of Electrical Engineering & Robotics
Keywords
robotics, robotic vision, computer vision, machine learning
Research field
Artificial intelligence, Electrical engineering
Field of Research code, Australian and New Zealand Standard Research Classification (ANZSRC), 2020
Qualifications
- PhD (Chemnitz University Of Technology)
Teaching
Professor Niko Suenderhauf is teaching into QUT's Master's of Robotics and AI, where he teaches and coordinates Robotic Vision (ENN583) and Advanced Machine Learning (ENN585).
He has been co-teaching and unit coordinating EGB339 (Introduction to Robotics) 2017-2022, and EGH419 (Mechatronics Design 3) 2018-2022. He was co-teaching EGH444 (Digital Signals and Image Processing) 2019-2021. Niko shared his expertise with the international community by giving three lectures at the 2019 International Summer School on Deep Learning for Robot Vision in Santiago, Chile.
Niko is General Chair of the Robotic Vision Summer School.
Experience
Research Project Leadership I am a Chief Investigator of the Australian Centre for Robotic Vision. In this role, I lead the project on Robotic Vision Evaluation and Benchmarking, and am deputy project leader for the Centre's Scene Understanding project. Robotic Vision Evaluation and Benchmarking (2018 – Present) Big benchmark competitions like ILSVRC or COCO fuelled much of the progress in computer vision and deep learning over the past years. We aim to recreate this success for robotic vision. To this end, we develop a set of new benchmark challenges for robotic vision that evaluate probabilistic object detection, scene understanding, uncertainty estimation, continuous learning for domain adaptation, continuous learning to incorporate previuosly unseen classes, active learning, and active vision. We combine the variety and complexity of real-world data with the flexibility of synthetic graphics and physics engines. See project
Scene Understanding and Semantic SLAM (2017 – Present) Making a robot understand what it sees is one of the most fascinating goals in my current research. To this end, we develop novel methods for Semantic Mapping and Semantic SLAM by combining object detection with simultaneous localisation and mapping (SLAM) techniques. We furthermore work on Bayesian Deep Learning for object detection, to better understand the uncertainty of a deep network’s predictions and integrate deep learning into robotics in a probabilistic way. See project
Bayesian Deep Learning and Uncertainty for Object Detection (2017 – Present) In order to fully integrate deep learning into robotics, it is important that deep learning systems can reliably estimate the uncertainty in their predictions. This would allow robots to treat a deep neural network like any other sensor, and use the established Bayesian techniques to fuse the network’s predictions with prior knowledge or other sensor measurements, or to accumulate information over time. We focus on Bayesian Deep Learning approaches for the specific use case of object detection on a robot in open-set conditions. See project
Reinforcement Learning for Robot Navigation and Complex Task Execution (2017 – Present) How can robots best learn to navigate in challenging environments and execute complex tasks, such as tidying up an apartment or assist humans in their everyday domestic chores? Often, hand-written architectures are based on complicated state machines that become intractable to design and maintain with growing task complexity. I am interested in developing learning-based approaches are effective and efficient, and scale better to complicated tasks. See project
Visual Place Recognition in Changing Environments (2012 – Present) An autonomous robot that operates on our campus should be able to recognize different places when it comes back to them after some time. This is important to support reliable navigation and localisation and therefore enable the robot to perform a useful task. The problem of visual place recognition gets challenging if the visual appearance of these places changed in the meantime. This usually happens due to changes in the lighting conditions (think day vs. night or early morning vs. late afternoon), shadows, different weather conditions, or even different seasons. We develop algorithms for vision-based place recognition that can deal with these changes in visual appearance. See project
Organised Research Workshops Dedicated workshops are a great way of getting in contact with fellow researchers from around the world that are working on similar scientific questions. Over the past years I was lead organiser or co-organiser for these workshops at leading international conferences:
- The Importance of Uncertainty in Deep Learning for Robotics (IROS 2019)
- Robotic Vision Probabilistic Object Detection Challenge (CVPR 2019)
- Deep Learning for Semantic Visual Navigation (CVPR 2019)
- New Benchmarks, Metrics, and Competitions for Robotic Learning (RSS 2018)
- Real-World Challenges and New Benchmarks for Deep Learning in Robotic Vision (CVPR 2018)
- Long-term autonomy and deployment of intelligent robots in the real-world (ICRA 2018)
- Learning for Localization and Mapping (IROS 2017)
- New Frontiers for Deep Learning in Robotics (RSS 2017)
- Deep Learning for Robotic Vision (CVPR 2017)
- Are the Sceptics Right? - Limits and Potentials of Deep Learning in Robotics (RSS 2016)
- Visual Place Recognition: What is it good for? (RSS 2016)
- Visual Place Recognition in Changing Environments (ICRA 2015)
- Visual Place Recognition in Changing Environments (CVPR 2015)
- Visual Place Recognition in Changing Environments (ICRA 2014)
- Robust and Multimodal Inference in Factor Graphs (ICRA 2013)
Publications
- Nicholson, L., Milford, M. & Suenderhauf, N. (2019). QuadricSLAM: Dual quadrics from object detections as landmarks in object-oriented SLAM. IEEE Robotics and Automation Letters, 4(1), 1–8. https://eprints.qut.edu.au/124209
- Garg, S., Suenderhauf, N. & Milford, M. (2022). Semantic-geometric visual place recognition: a new perspective for reconciling opposing views. International Journal of Robotics Research, 41(6), 573–598. https://eprints.qut.edu.au/133595
- Suenderhauf, N., Dayoub, F., Hall, D., Skinner, J., Zhang, H., Carneiro, G. & Corke, P. (2019). A probabilistic challenge for object detection. Nature Machine Intelligence, 1(9). https://eprints.qut.edu.au/132632
- Suenderhauf, N., Brock, O., Scheirer, W., Hadsell, R., Fox, D., Leitner, J., Upcroft, B., Abbeel, P., Burgard, W., Milford, M. & Corke, P. (2018). The limits and potentials of deep learning for robotics. International Journal of Robotics Research, 37(4 - 5), 405–420. https://eprints.qut.edu.au/121238
- Bruce, J., Suenderhauf, N., Mirowski, P., Hadsell, R. & Milford, M. (2018). Learning deployable navigation policies at kilometer scale from a single traversal. Proceedings of Machine Learning Research (PMLR), Volume 87: Conference on Robot Learning 2018, 346–361. https://eprints.qut.edu.au/124208
- Anderson, P., Wu, Q., Teney, D., Bruce, J., Johnson, M., Suenderhauf, N., Reid, I., Gould, S. & Van Den Hengel, A. (2018). Vision-and-Language Navigation: Interpreting visually-grounded navigation instructions in real environments. Proceedings of the 30th IEEE/CVF Conference on Computer Vision and Pattern Recognition, 3674–3683. https://eprints.qut.edu.au/124633
- Suenderhauf, N., Pham, T., Latif, Y., Milford, M. & Reid, I. (2017). Meaningful maps with object-oriented semantic mapping. Proceedings of the 2017 IEEE/RSJ International Conference on Intelligent Robots and Systems (IROS 2017), 5079–5085. https://eprints.qut.edu.au/130279
- Lowry, S., Suenderhauf, N., Newman, P., Leonard, J., Cox, D., Corke, P. & Milford, M. (2016). Visual place recognition: A survey. IEEE Transactions on Robotics, 32(1), 1–19. https://eprints.qut.edu.au/105651
- Suenderhauf, N., Shirazi, S., Dayoub, F., Upcroft, B. & Milford, M. (2015). On the performance of ConvNet features for place recognition. Proceedings of the 2015 IEEE/RSJ International Conference on Intelligent Robots and Systems (IROS 2015), 4297–4304. https://eprints.qut.edu.au/101053
- Suenderhauf, N. & Protzel, P. (2012). Switchable constraints for robust pose graph SLAM. Proceedings of the 2012 IEEE/RSJ International Conference on Intelligent Robots and Systems (IROS 2012), 1879–1884. https://eprints.qut.edu.au/109582
QUT ePrints
For more publications by Niko, explore their research in QUT ePrints (our digital repository).
Awards
- Type
- Academic Honours, Prestigious Awards or Prizes
- Reference year
- 2020
- Details
- Amazon Research Award for the project "Learning Robotic Navigation and Interaction from Object-based Semantic Maps". This internationally competitive and prestigious award supports my research towards intelligent robots operating alongside humans in domestic environments with $120,000AUD.
- Type
- Academic Honours, Prestigious Awards or Prizes
- Reference year
- 2018
- Details
- Google Faculty Research Award for the project "The Large Scale Robotic Vision Perception Challenge". This award "recognises and supports world-class faculty pursuing cutting-edge research". My proposal was selected after expert reviews out of 1033 proposals from 360 universities in 46 countries. The acceptance rate was only 14.7%.The award sum of over $74,000AUD supported my research activities of creating new robotic vision research competitions for the international community.
- Type
- Advisor/Consultant for Community
- Reference year
- 2019
- Details
- I am one of two chairs for the International Technical Committee for Computer and Robot Vision of the Institute of Electrical and Electronics Engineers (IEEE). In this role, I oversee and steer the organisation of events and activities for the international research community alongside my co-chair, Prof Scaramuzza from ETH Zurich.
- Type
- Editorial Role for an Academic Journal
- Reference year
- 2019
- Details
- I was invited to be a Member of the Editorial Board of the International Journal of Robotics Research (IJRR), the highest-impact journal in robotics, alongside full professors from institutions such as Uni of Oxford, Stanford, MIT, or Harvard. From 2015-2019 I served as associate editor of the IEEE Robotics and Automation Letters journal.
- Type
- Editorial Role for an Academic Journal
- Reference year
- 2018
- Details
- Guest Editor for Special Issue on "Deep Learning for Robotic Vision" with the leading Q1 journal International Journal on Computer Vision (IJCV)
- Type
- Editorial Role for an Academic Journal
- Reference year
- 2017
- Details
- Coordinating Guest Editor for Special Issue on Deep Learning for Robotics with leading Q1 journal in robotics: International Journal of Robotics Research (IJRR)
- Type
- Academic Honours, Prestigious Awards or Prizes
- Reference year
- 2015
- Details
- QUT Vice Chancellor's Performance Award
- Type
- Editorial Role for an Academic Journal
- Reference year
- 2015
- Details
- Associate Editor for IEEE Robotics and Automation Letters (RA-L) Journal since 2015
Selected research projects
- Title
- ARC Centre of Excellence for Robotic Vision (ACRV)
- Primary fund type
- CAT 1 - Australian Competitive Grant
- Project ID
- CE140100016
- Start year
- 2014
- Keywords
- Robotic Vision; Robotics; Computer Vision
Projects listed above are funded by Australian Competitive Grants. Projects funded from other sources are not listed due to confidentiality agreements.
Supervision
Current supervisions
- Solving Manipulation Tasks With Implicit Neural Representations
PhD, Principal Supervisor
Other supervisors: Dr Feras Dayoub - Utilising cooperation and exploration techniques to solve complex, multi-stage reinforcement learning tasks
PhD, Associate Supervisor
Other supervisors: Dr Chris Lehnert, Distinguished Emeritus Professor Peter Corke - Weakly supervised segmentation of underwater imagery
PhD, Associate Supervisor
Other supervisors: Dr Frederic Maire, Dr Ross Marchant
Completed supervisions (Doctorate)
Supervision topics
- Mapping the world: understanding the environment through spatio-temporal implicit representations
- Semantic SLAM for robotic scene understanding, geometric-semantic representations for infrastructure monitoring and maintenance
- Implicit representations for place recognition and robot localisation
- Robot learning for navigation, interaction, and complex tasks
- Augmented reality (AR) applications for robotic scene understanding
- Adaptive and efficient robot positioning