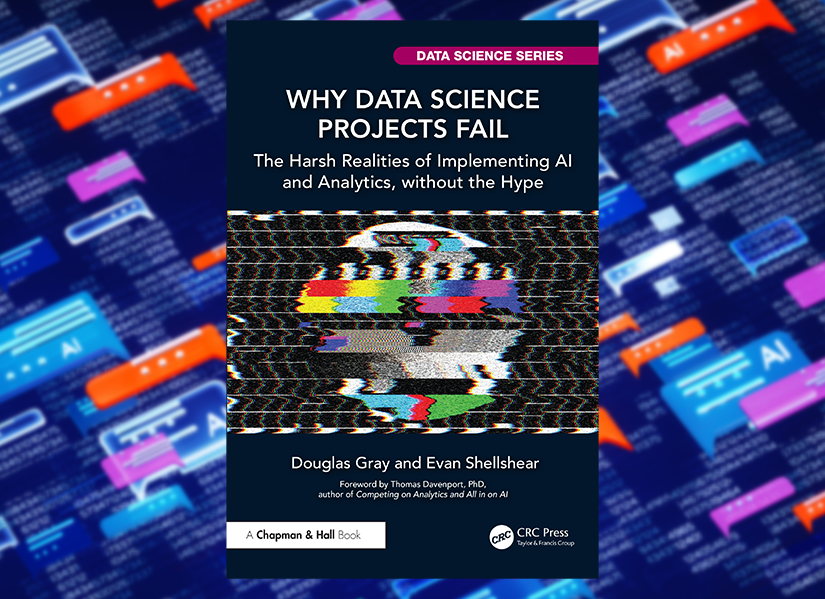
Dr Evan Shellshear, 18 September, 2024
The promise of data science has captivated organisations across various industries, luring them with the potential of uncovering hidden insights, optimising operations, and improvements to the bottom line. Yet, despite the growing investment in data science initiatives, a staggering number of projects fail to deliver on their promises. The question is, why?
The brand new book, Why Data Science Projects Fail by QUT Adjunct Professor, Dr Evan Shellshear and his co-author Doug Gray, uncovers the disconcerting reality that a significant portion of data science projects do not meet their intended goals. According to the research, failure rates of 80% are common, but even these numbers belie a more concerning truth. These figures underscore a pervasive problem that cannot be ignored: data science projects are failing at an alarming rate, and this has serious implications for businesses, researchers, and policymakers alike.
20 Years of frameworks and research: what do we have to show for it?
One of the central themes in the book is the inherent complexity of data science projects. Unlike traditional IT projects, data science initiatives involve navigating complex mathematics, unique people challenges as well as often unreasonable expectations, where the path to success is often obscured by numerous uncertainties. To address these challenges, existing frameworks for IT have been extended over the last two decades but according to the research, these are yet to put a dent in the failure rate. So what to do?
The first step is to understand the types of failures, and to do this, the authors put them into four categories:
- Strategy
- Process
- People
- Technology
Then the authors look at whether the failures are something afflicting so-called analytically mature organisations (i.e. ones that consistently deliver data science projects successfully) or analytically immature organisations. By looking at the data this way, the authors reveal the shocking truth that the real failure rate for most organisations (which are analytically immature) is over 90% and that for analytically mature organisations is closer to 40%.
Learning from Failure: The Importance of Post-Mortem Analyses
Despite the grim statistics, the book emphasises that failure is not the end—it is a learning opportunity. Post-mortem analyses are crucial for understanding what went wrong and how future projects can be improved.
The book shows that learning from our failures is one of the most effective ways to learn and so presents many anonymised stories from their network of AI failures to let the reader peer behind the corporate walls and reveal disastrous data science projects which normally never escape the communication confines of the corporation. By revealing stories from inside the organisation, the reader is able to draw much richer conclusions than superficial analyses conducted from the outside, lacking the detailed facts.
The stories are chosen to illustrate the most important themes revealed by the research. Although dozens of reasons for failure were discovered, there were a small number of repeating issues that the book explores in detail and the stories related to these failures help the reader contextualise and avoid such problems in their own projects.
A Call to Action: Transforming the Data Science Landscape
Why Data Science Projects Fail is not just a diagnosis of a widespread problem; it is a call to action. The book outlines several strategies that organisations can adopt to improve their data science outcomes, which we summarise here:
- Strategy: Find those strategically important projects for which data science and business analytics can help accelerate the strategy.
- Process: Make sure you’ve got the right data to solve the problem at hand and don’t expect any miracles if your data is no good.
- People: If you don’t have the right people or people don’t support an analytics approach, then don’t even try.
- Technology: Invest in the right types of tools to solve problems that are known to have solutions you can implement.
These recommendations are based on the most important failure reasons in each theme and what is required to address them. The book delves into each of these topics in much further detail pointing the reader to the practical frameworks that the authors have found help them, instead of untested academic theories.
Why This Book Matters
Why Data Science Projects Fail is a timely and necessary exploration of a critical issue facing the data science community. As organisations continue to invest in data-driven initiatives, the lessons outlined in this book will be invaluable for avoiding the pitfalls that have plagued so many projects. For anyone involved in data science—whether as a practitioner, manager, or executive—this book is an essential read. It not only highlights the dangers of ignoring the challenges inherent in data science but also provides a roadmap for overcoming them and achieving success in this rapidly evolving field.
Most importantly, this book forms the core of QUT’s AI for Business Value: Microcredential, which I co-facilitate. This microcredential helps learners understand where AI investments make most sense and can have the greatest impact, ensuring organisations are ready to face a future powered by AI.