Supervisors
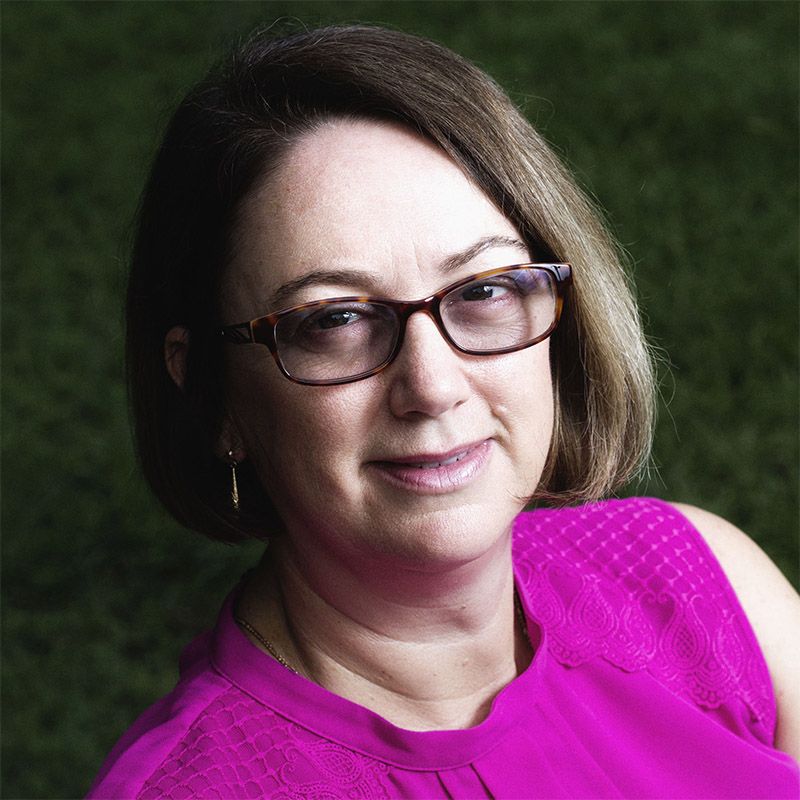
- Position
- Professor
- Division / Faculty
- Faculty of Health
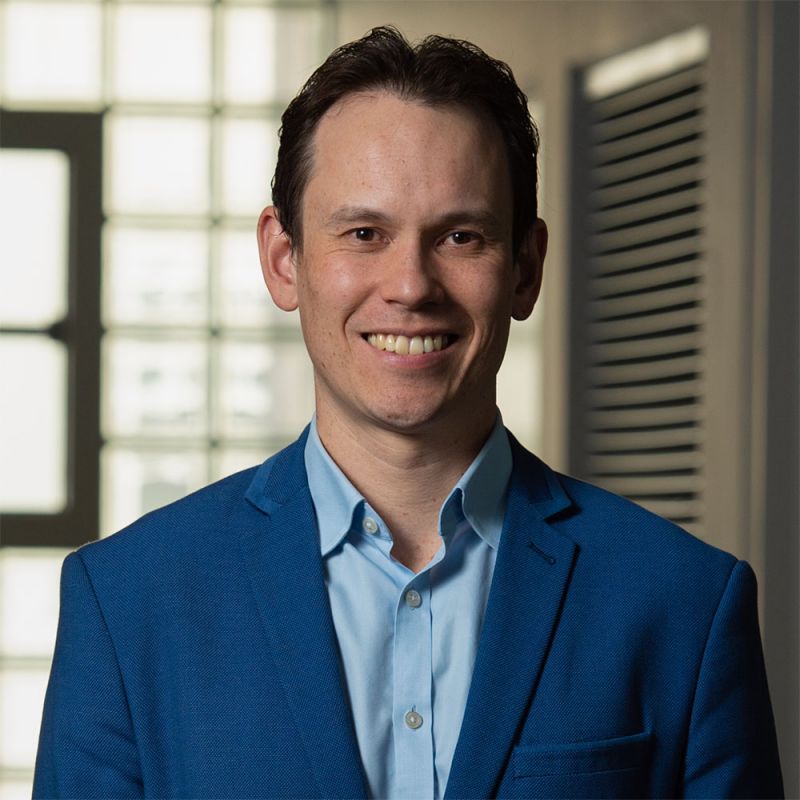
- Position
- Professor in Electrical Engineering
- Division / Faculty
- Faculty of Engineering
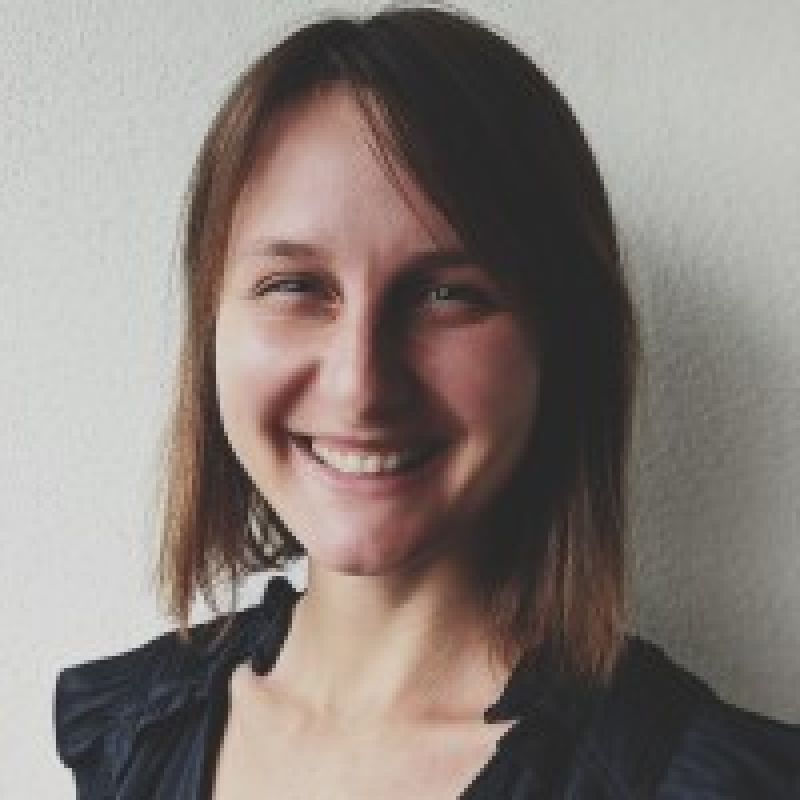
- Position
- Allergy Research Postdoctoral Fellow
- Division / Faculty
- Faculty of Health
Overview
https://research.qut.edu.au/ciic/https://research.qut.edu.au/ciic/We're seeking a motivated student to join the multidisciplinary project that brings together computer vision and deep learning field with pollen aerobiology. This is a fully funded PhD program for a three-year period starting in 2024. It's part of the project funded by the Australian Research Council Discovery Program—Digitally-Integrated Smart Sensing of Diverse Airborne Grass Pollen Sources. The successful candidate will be primarily based in the Allergy Research Group at QUT's Kelvin Grove campus.
Grass pollen is the main outdoor allergen source globally, triggering hayfever and asthma in over 500 million people. With over 10,000 species, the influence of grass type, location and climate on pollen in the air is not yet known. The temporal and spatial relationships between different grass pollen sources and airborne pollen loads are poorly defined, making airborne grass pollen forecasts imprecise. This is a key issue since grasses from subtropical and temperate climate zones differ in response to environmental factors. The project aims to use artificial intelligence on digital camera images to learn to see local grass flowers and integrate this information with air sensors trained to detect grass pollen types.
Research activities
- Assist with digital camera monitoring and collecting data on field observations (using iNaturalist) at pollen monitoring sites.
- Develop, apply and evaluate deep learning models to recognise flowering of grasses of subtropical and temperate subfamilies using near-ground level digital cameras.
- Collaborate with interdisciplinary research teams from QUT, Macquire University and MeteoSwiss (Swiss Federal Office of Meteorology and Climatology).
- Contribute to the preparation of research publications and reports.
Outcomes
The successful candidate will contribute to advancing the capabilities to track airborne grass pollen types. These outcomes can transform how pollen can be monitored to reduce the health and economic burden of allergies.
Skills and experience
Essential skills and experience
- Background in computer vision and machine learning
- A research degree
- Excellent written and oral communication skills
- Some knowledge of a programming language, e.g. Python/R
Desirable computer vision and machine learning skills
- Technical experience with image processing tasks such as detection, recognition, segmentation, semi-supervised annotation and data augmentation (in ecology, aerial imagery, medical imagery or related domains)
- Technical experience with machine learning software packages for these or similar tasks, preferably recent state of the art approaches
- Technical experience in curating large, rich and sometimes unevenly populated datasets comprising a mixture of imagery and metadata.
Experience and/or interest in ecology and/or allergy research is also beneficial.
Keywords
Contact
If you require more information or you'd like to apply for this opportunity, please email your expression of interest—including your résumé and a copy of your academic transcripts—to Dr Andelija Milic. We'll build a shortlist of potentially suitable applicants at the beginning of 2024.