Supervisors
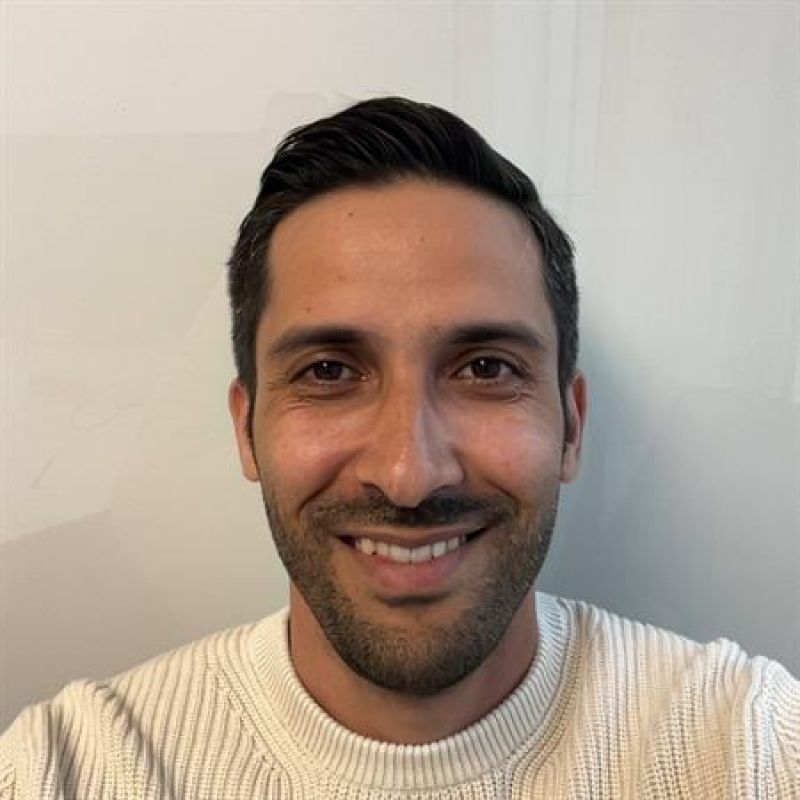
- Position
- Senior Lecturer in Statistical Data Science
- Division / Faculty
- Faculty of Science
Overview
Forecasting renewable energy production is crucial for ensuring stable and sustainable energy grids. Traditional approaches often involve a two-stage process: first, energy production forecasts are generated, then decisions, such as how much energy to produce from various sources (wind, solar, fossil fuels), are made based on those forecasts. This disjointed process, where forecast accuracy and decision-making optimization are treated separately, can lead to sub-optimal outcomes due to conflicting objective functions.
The goal of this project is to bridge these stages by focusing on decision-informed forecasting, where decisions and their impact are directly integrated into the forecasting model using Bayesian methods. This Bayesian-focused learning framework will not only generate probabilistic forecasts but will also directly optimize these forecasts based on the eventual decisions they support. The result is improved alignment between energy production decisions and desired outcomes, such as price stability or sustainability targets.
Research activities
This project will develop Bayesian-based probabilistic forecasting models that incorporate decision-making processes, optimizing for both forecast accuracy and decision outcomes simultaneously. The key activities include:
- Bayesian modeling for forecasting
- We will use Bayesian methods to develop probabilistic models that account for the uncertainty in renewable energy production (e.g. wind, solar). These models will be designed for high-frequency data (such as 5-minute intervals) to support operational decision-making.
- Decision-optimised learning
- By directly incorporating decision variables into the forecasting process, the models will optimise outcomes such as energy load balance, pricing, and sustainability goals, avoiding sub-optimal results that occur when decisions are based on average forecast accuracy alone.
- Iterative learning
- As new data becomes available, Bayesian updating will be used to refine and improve both the forecasts and decision-making outcomes, ensuring that the system adapts dynamically to changing energy patterns and decision constraints.
Outcomes
This main objective and expected outcomes of this project are as follows:
- Optimal decision-making
- By directly incorporating decision variables into the forecasting models, the expected outcome is that energy production decisions (e.g. how much to produce from wind, solar, or fossil fuels) will be optimized. This integration will align forecast outcomes with real-world decision objectives such as energy load balance, cost efficiency, and sustainability targets.
- Reduction in forecasting and decision-making gaps
- Traditional methods that separate forecasting and decision-making can result in suboptimal outcomes due to the misalignment of objectives. By using a Bayesian decision-focused approach, the gap between forecast accuracy and decision quality will be significantly reduced. This will lead to better decision outcomes even if the forecast accuracy is not perfect in traditional terms.
- Improved energy grid stability
- The project is expected to contribute to the stability and efficiency of the energy grid by enabling more accurate and relevant energy production forecasts. Optimising energy output based on integrated decision variables will help to ensure a more balanced energy grid, reducing overproduction and underproduction risks.
Skills and experience
- Deep understating of Bayesian modeling.
- Fluent in R/Python.
- Familiar with statistical modeling.
Scholarships
You may be eligible to apply for a research scholarship.
Explore our research scholarships
Keywords
Contact
Contact the supervisor for more information.