Supervisors
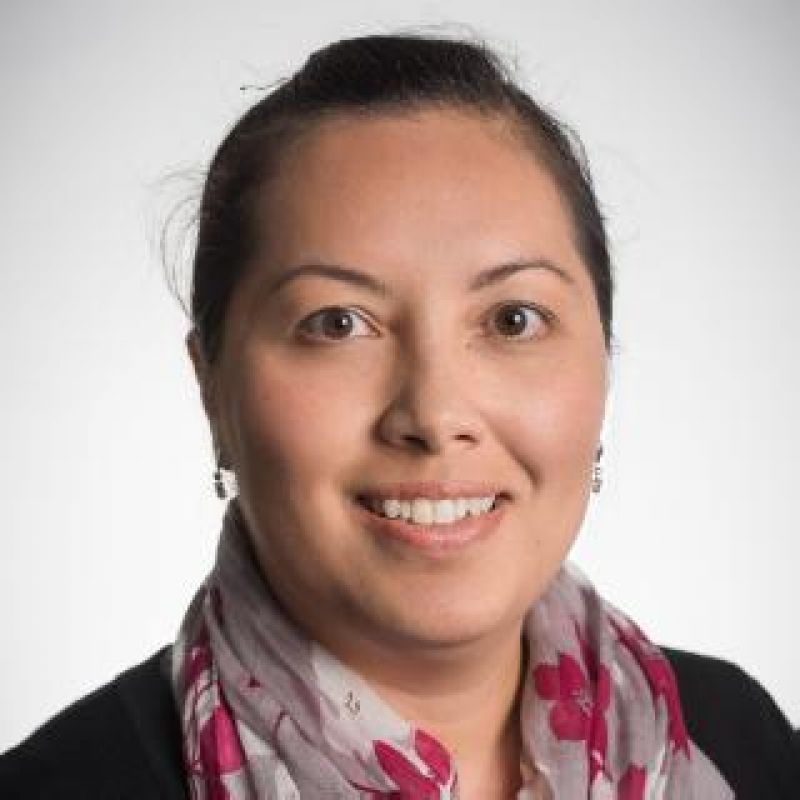
- Position
- Associate Professor in Statistics
- Division / Faculty
- Faculty of Science
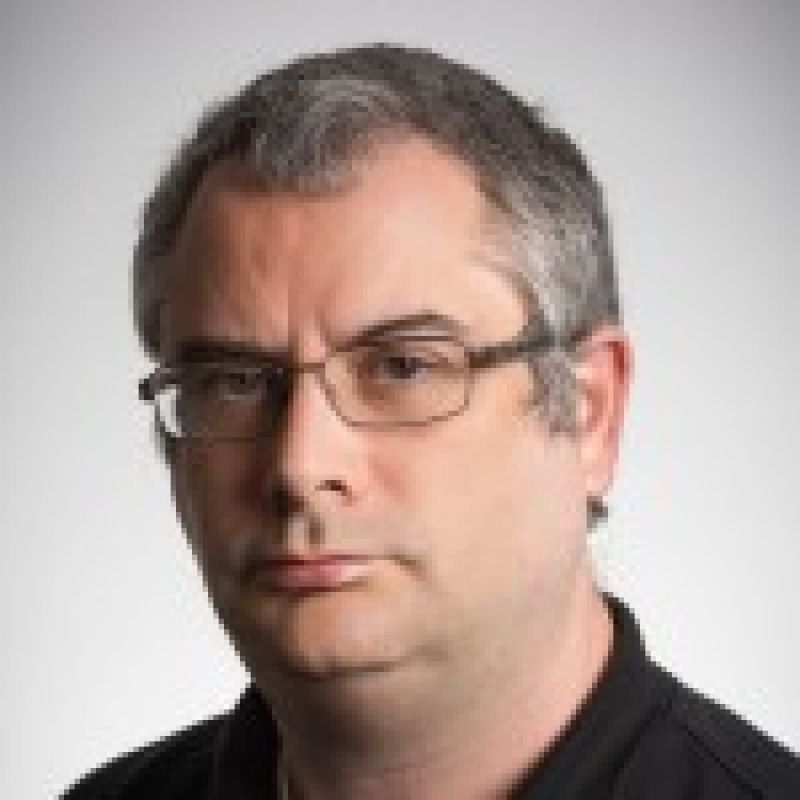
- Position
- Associate Professor in Data Science and Government Statistics Chair (acting)
- Division / Faculty
- Faculty of Science
External supervisors
- Edwin Lu, Australian Bureau of Statistics
Overview
The Australian Bureau of Statistics (ABS) conducts surveys to collect information from individuals, households and businesses in order to produce statistics and data products to help inform decision-making. Unlike a census, in which an entire population of interest is enumerated (e.g., all individuals residing in Australia), a survey collects information from only a sample (subset) of a population of interest. Estimators are then used to estimate quantities related to the population of interest using information from the sample. Currently, the generalised regression (GREG) estimator is commonly used in the ABS, as it allows more than one auxiliary variable to assist with estimation and thus is often quite efficient (i.e., has low mean square error).
It is implicit in the GREG estimator that the target variable of interest (for which we want to estimate a population quantity for, such as a total or a mean) is modelled as having a linear relationship with auxiliary variables or their univariate transformations. There is potential to improve from the GREG estimator by developing estimators that allow the target variable of interest to have a nonlinear relationship with auxiliary variables or their univariate transformations. Such nonlinear relationships can be modelled using techniques from fields such as functional data analysis and machine learning. It may be possible to incorporate these techniques into survey estimation in various ways, such as by:
- directly modelling the target variable of interest in terms of auxiliary variables and aggregating the unit-level predictions while adjusting for prediction error, or
- using the aggregate from the predictions as a benchmark for the GREG estimator, or
- modelling the auxiliary variables to have them reflected in the probability of inclusion/missingness, or
- using them to select auxiliary variables for the GREG estimator.
More generally, rapid developments in the field of machine learning creates opportunities for machine learning to assist with surveys potentially in other ways.
Research activities
The ABS Methodology and Data Science Division (MDSD) would like to explore how techniques from functional data analysis, machine learning and possibly other areas of statistics can assist with surveys. Research problems include:
- Find conditions under which m-assisted estimators (where m is a nonlinear model from functional data analysis, machine learning or possibly other areas of statistics) are more efficient (i.e., have lower mean square error) than GREG estimators.
- Find conditions under which m-assisted estimators, together with Rao-Blackwellisation, are more efficient than GREG estimators. Investigate how much of the gain in efficiency is due to model m and how much is due to Rao-Blackwellisation.
- Explore if machine learning can be used to select auxiliary variables for GREG estimators.
- More generally, explore how nonlinear models (e.g., from functional data analysis or machine learning) can assist with surveys.
Outcomes
This is a PhD-level project, so the expectation is a completed thesis (either by monograph or publication) worthy of the award of a PhD.
Skills and experience
Experience in statistical methods and modelling, knowledge of survey sampling methods, and knowledge of machine learning or other modelling methods.
Scholarships
You may be eligible to apply for a research scholarship.
Explore our research scholarships
Keywords
Contact
Contact the Supervisor for more information