Supervisors
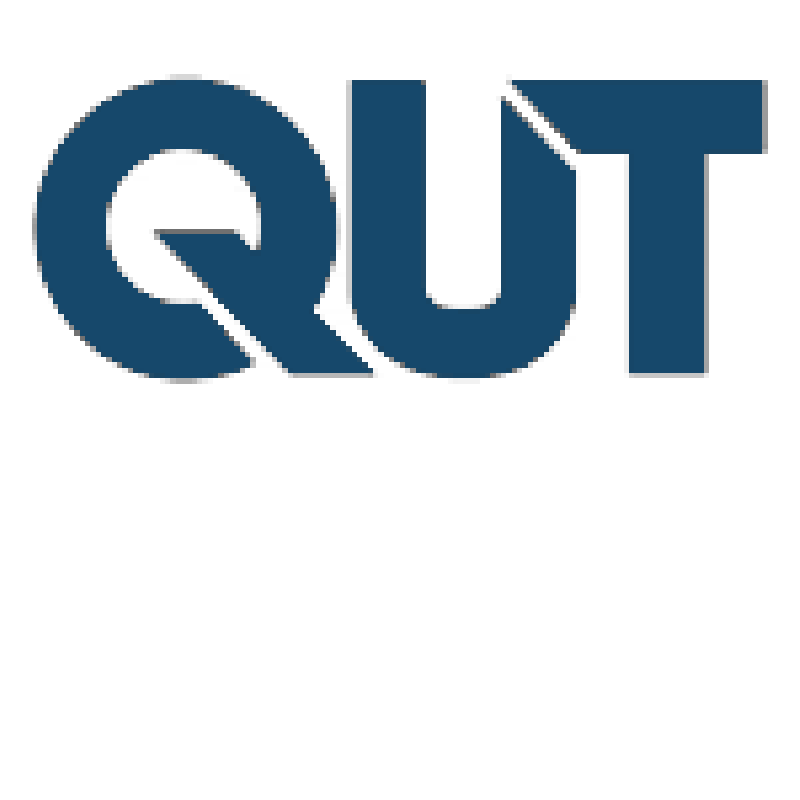
- Position
- Division / Faculty
Overview
In the age of Big Data, machine learning methods, and modern statistics the adage "all models are wrong but some are useful" has never been so true. This project will investigate data science approaches where more than one model makes sense for the data. Is it better to choose a single model or is there something to be gained from multiple models?
This project will look at variable selection methods, penalised regression, Bayesian model averaging and conformal prediction. The research has the potential to improve statistical and machine learning methods that are widely used and offer new insights to data science problems.
This topic could be developed into a VRES or Honours level project.
Research activities
Research activities include:
- regularly meeting and discuss the project with Dr Matt Sutton
- learning about model selection, model averaging and conformal prediction
- comparing existing methods
- developing and implementing new methods using synthetic data.
Outcomes
Potential outcomes include:
- Code for model selection, averaging and prediction.
- Comparison of different approaches.
- Potential coauthorship on a research article.
Skills and experience
Recommended MXB242 (regression and design), some experience in programming (R preferred). Some experience with Bayesian methods is desirable but not required.
Scholarships
You may be eligible to apply for a research scholarship.
Explore our research scholarships
Keywords
- stochatic modelling
- computational statistics
- data science
- machine learning
- Bayesian
- uncertainty quantification
- variable selection
Contact
Contact the supervisor for more information.