Supervisors
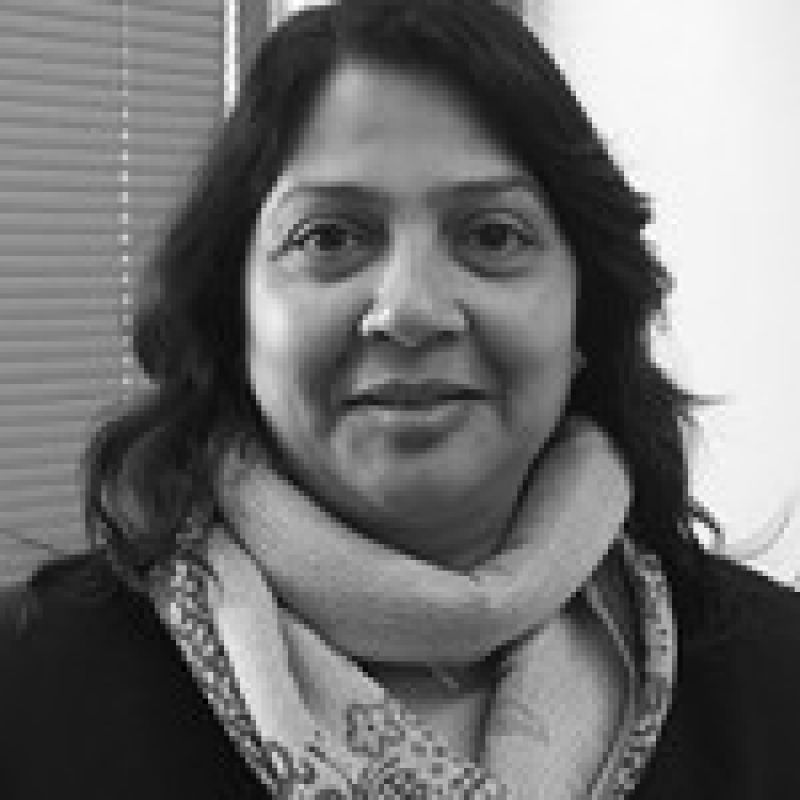
- Position
- Professor
- Division / Faculty
- Faculty of Science
Overview
In deep learning models, language models and word embedding methods have become popular to understand the context of text data. Popular language models such as BERT have limitations in terms of the token length. There exist some corpora that have longer text with an average of 1000 tokens. Additionally, these corpora are text-heavy and only include some images.
In our prior works, we have developed several multi-modality models on social media datasets.
Research activities
This project will introduce you to the hot topic of language models and the fields of natural language processing and text mining.
In this project, your task will be to provide different feature representation methods (e.g. text: fasttext, word2vect, glove and image: VGG19, ResNet) and fusion methods (e.g. early, late, cross-attention, gated module) on these datasets. You will be provided with all relevant codes and datasets.
Your task will be to conduct systematic experiments to explore the text-heavy multi-modal datasets (e.g. Yelp) and report the findings to compare various feature representation and fusion methods.
Outcomes
Outcomes include:
- a report describing experiments and their outcomes
- a code pipeline integrating all models that will be experimented
- an academic paper sharing the empirical analyses.
Skills and experience
- Python
- machine learning
Scholarships
You may be eligible to apply for a research scholarship.
Explore our research scholarships
Keywords
Contact
Contact the supervisor for more information.