Supervisors
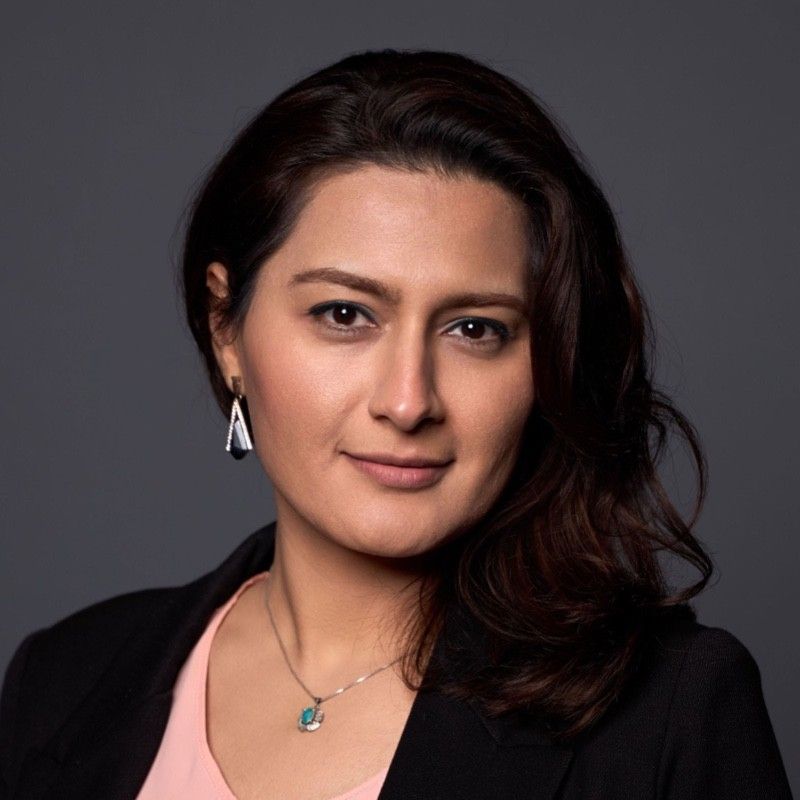
- Position
- Lecturer
- Division / Faculty
- Faculty of Engineering
Overview
Gastro-intestinal (GI) cancers affect over 28 million patients annually, representing about 26% of the global cancer incidence and 35% of all cancer-related deaths (American Cancer Society, 2018). Besides, GI cancer is the second deadliest cancer type with reported 3.4 million GI related deaths globally in 2018.
Endoscopy plays a crucial role in diagnosing and managing GI disorders by providing detailed internal anatomical visualisation. Endoscopic procedures have become a cornerstone in minimally invasive surgery, offering patients reduced trauma and quicker recovery times. In this context, accurate and dynamic 3D reconstruction of the endoscopic scene is critical to enhancing the surgeon’s spatial understanding and navigation, facilitating more precise and efficient interventions. However, the complex and constrained nature of endoscopic scenes poses significant challenges for traditional 3D reconstruction techniques due to factors such as limited field-of-view, occlusions, and dynamic tissue deformation. Recent advancements in deep neural networks and their adoption for 3D reconstruction from endoscopic videos has shown promising paths. This project aims to integrate Simultaneous Localisation and Mapping (SLAM) with advanced deep learning techniques to enhance their capabilities.
Research engagement
- Develop integrated algorithms for seamless endoscopy camera pose estimation and 3D organ reconstruction.
- Assess computational feasibility for clinical deployment and compare with baseline methods in terms of accuracy and efficiency.
- Validate using synthetic and real endoscopy datasets to ensure robustness and reliability.
Outcomes
- Achieve high-fidelity, 3D models of gastrointestinal organs from endoscopic videos.
- Provide accurate navigation during endoscopic procedures.
Skills and experience
- Strong programming background (preferably in Python).
- Machine learning experience preferred but not mandatory.
- Understanding of computer vision and deep learning concepts is beneficial.
Start date
1 November, 2024End date
28 February, 2025Location
School of Electrical Engineering and Robotics
Keywords
- AI in medical imaging
- Deep learning
- Computer vision
- Machine learning
- 3D reconstruction
- Robotics in healthcare
Contact
maryam.haghighat@qut.edu.au