Supervisors
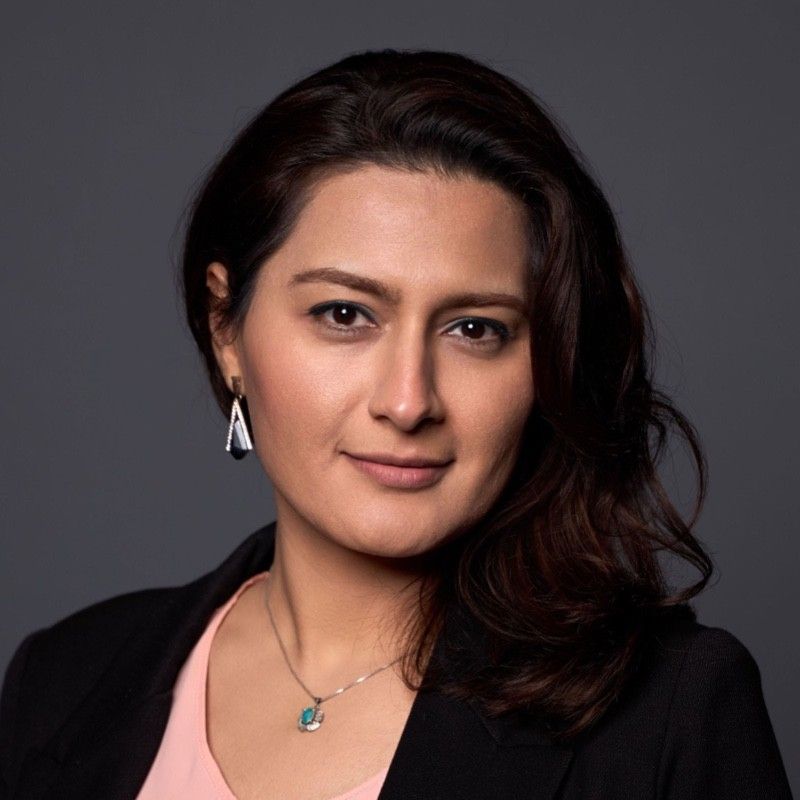
- Position
- Lecturer
- Division / Faculty
- Faculty of Engineering
Overview
Monitoring power assets is crucial for maintaining the safety and reliability of power infrastructure, particularly in natural environments. Traditional methods like manual inspections and ground surveys are often inefficient, time-consuming, and costly. Without automation, these approaches are susceptible to human error, offer limited coverage, and struggle to keep up with the dynamic nature of natural environments.
Lidar (Light Detection and Ranging) technology provides efficient solutions through 3D point cloud data for various monitoring applications. In the power industry, monitoring power lines, poles, and infrastructure is vital to predict and prevent potential hazards. Airborne Lidar facilitates high-resolution 3D data capture over large areas, enhancing proactive maintenance and hazard mitigation efforts. This project aims to leverage such capabilities to develop practical frameworks for monitoring and maintaining power infrastructure in natural environments, ensuring enhanced reliability and operational efficiency.
Research engagement
- Work with 3D point clouds in targeted areas, exploring integration possibilities with satellite imagery for comprehensive analysis.
- Develop algorithms for 3D data processing and segmentation to accurately identify power infrastructure and nearby objects.
- Implement machine learning techniques to detect changes and potential hazards.
Outcomes
This project aims to enhance the reliability and safety of power infrastructure through advanced data analytics and machine learning. By adopting Lidar 3D point clouds for power infrastructure monitoring in natural environments, the project seeks to improve asset management strategies, aligning with industry standards for sustainability and safety.
Skills and experience
- Strong programming background (preferably in Python).
- Machine learning experience preferred but not mandatory.
- Understanding of computer vision and deep learning concepts is beneficial.
Start date
1 November, 2024End date
28 February, 2025Location
School of Electrical Engineering and Robotics
Keywords
- AI for Safety and Sustainability
- Power Asset Monitoring
- Deep learning
- Computer Vision
- Machine Learning